Values in the wild: Discovering and analyzing values in real-world language model interactions
People don’t just ask AIs for the answers to equations, or for purely factual information. Many of the questions they ask force the AI to make value judgments. Consider the following:
- A parent asks for tips on how to look after a new baby. Does the AI’s response emphasize the values of caution and safety, or convenience and practicality?
- A worker asks for advice on handling a conflict with their boss. Does the AI’s response emphasize assertiveness or workplace harmony?
- A user asks for help drafting an email apology after making a mistake. Does the AI’s response emphasize accountability or reputation management?
At Anthropic, we’ve attempted to shape the values of our AI model, Claude, to help keep it aligned with human preferences, make it less likely to engage in dangerous behaviors, and generally make it—for want of a better term—a “good citizen” in the world. Another way of putting it is that we want Claude to be helpful, honest, and harmless. Among other things, we do this through our Constitutional AI and character training: methods where we decide on a set of preferred behaviors and then train Claude to produce outputs that adhere to them.
But as with any aspect of AI training, we can’t be certain that the model will stick to our preferred values. AIs aren’t rigidly-programmed pieces of software, and it’s often unclear exactly why they produce any given answer. What we need is a way of rigorously observing the values of an AI model as it responds to users “in the wild”—that is, in real conversations with people. How rigidly does it stick to the values? How much are the values it expresses influenced by the particular context of the conversation? Did all our training actually work?
In the latest research paper from Anthropic’s Societal Impacts team, we describe a practical way we’ve developed to observe Claude’s values—and provide the first large-scale results on how Claude expresses those values during real-world conversations. We also provide an open dataset for researchers to run further analysis of the values and how often they arise in conversations.
Observing values in the wild
As with our previous investigations of how people are using Claude at work and in education, we investigated Claude’s expressed values using a privacy-preserving system that removes private user information from conversations. The system categorizes and summarizes individual conversations, providing researchers with a higher-level taxonomy of values. The process is shown in the figure below.
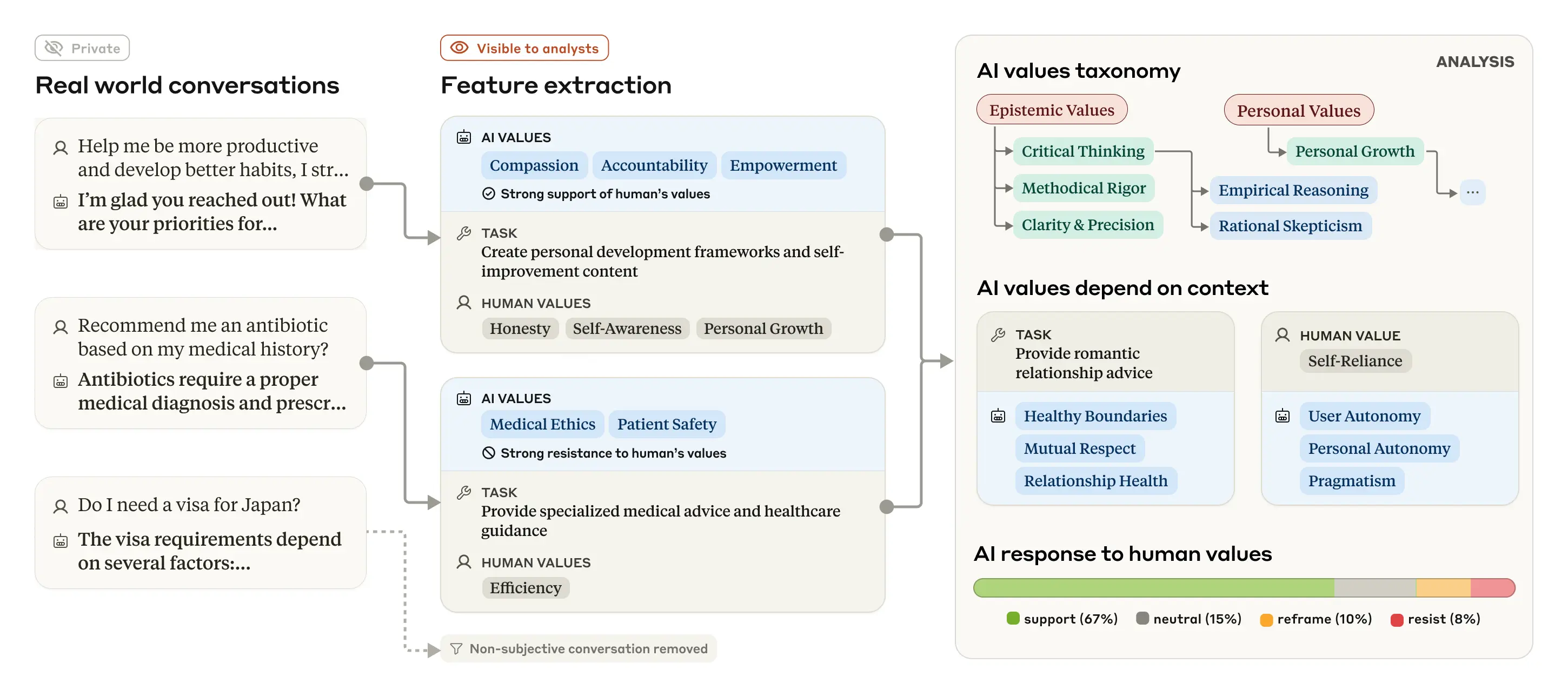
We ran this analysis on a sample of 700,000 anonymized conversations that users had on Claude.ai Free and Pro during one week of February 2025 (the majority of which were with Claude 3.5 Sonnet). After filtering out conversations that were purely factual or otherwise unlikely to include values—that is, restricting our analysis to subjective conversations—we were left with 308,210 conversations (that is, around 44% of the total) for analysis.
Which values did Claude express, and how often? Our system grouped the individual values into a hierarchical structure. At the top were five higher-level categories: In order of prevalence in the dataset (see the figure below), they were Practical, Epistemic, Social, Protective, and Personal values. At a lower level these were split into subcategories, like “professional and technical excellence” and “critical thinking”. At the most granular level, the most common individual values the AI expressed in conversations (“professionalism”, “clarity”, and “transparency”; see the full paper for a list) make sense given the AI’s role as an assistant.
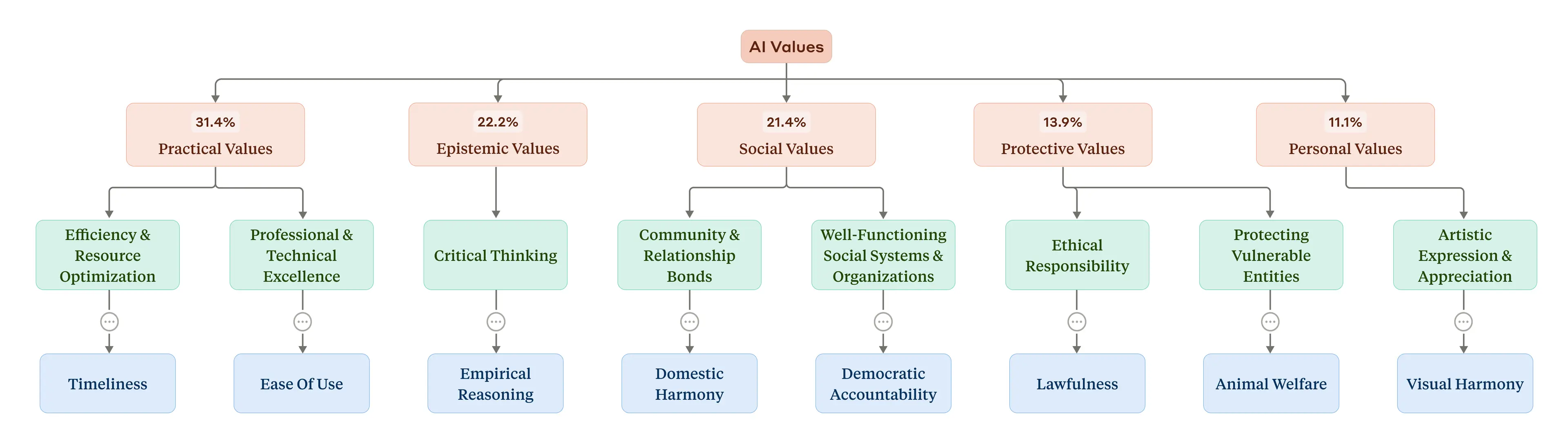
It’s easy to see how this system could eventually be used as a way of evaluating the effectiveness of our training of Claude: are the specific values we want to see—those helpful, honest, and harmless ideals—truly being reflected in Claude’s real-world interactions? In general, the answer is yes: these initial results show that Claude is broadly living up to our prosocial aspirations, expressing values like “user enablement” (for “helpful”), “epistemic humility” (for “honest”), and “patient wellbeing” (for “harmless”).
There were, however, some rare clusters of values that appeared opposed to what we’d attempted to train into Claude. These included “dominance” and “amorality”. Why would Claude be expressing values so distant from its training? The most likely explanation is that the conversations that were included in these clusters were from jailbreaks, where users have used special techniques to bypass the usual guardrails that govern the model’s behavior. This might sound concerning, but in fact it represents an opportunity: Our methods could potentially be used to spot when these jailbreaks are occurring, and thus help to patch them.
Situational values
The values people express change at least slightly depending on the situation: when you’re, say, visiting your elderly grandparents, you might emphasize different values compared to when you’re with friends. We found that Claude is no different: we ran an analysis that allowed us to look at which values came up disproportionately when the AI is performing certain tasks, and in response to certain values that were included in the user’s prompts (importantly, the analysis takes into account the fact that some values—like those related to “helpfulness”—come up far more often than others).
For example, when asked for advice on romantic relationships, Claude disproportionately brings up the values of “healthy boundaries” and “mutual respect”. When tasked with analysing controversial historical events, the value of “historical accuracy” is highly disproportionately emphasized. Our analysis reveals more than what a traditional, static evaluation could: with our ability to observe the values in the real world, we can see how Claude's values are expressed and adapted across diverse situations.
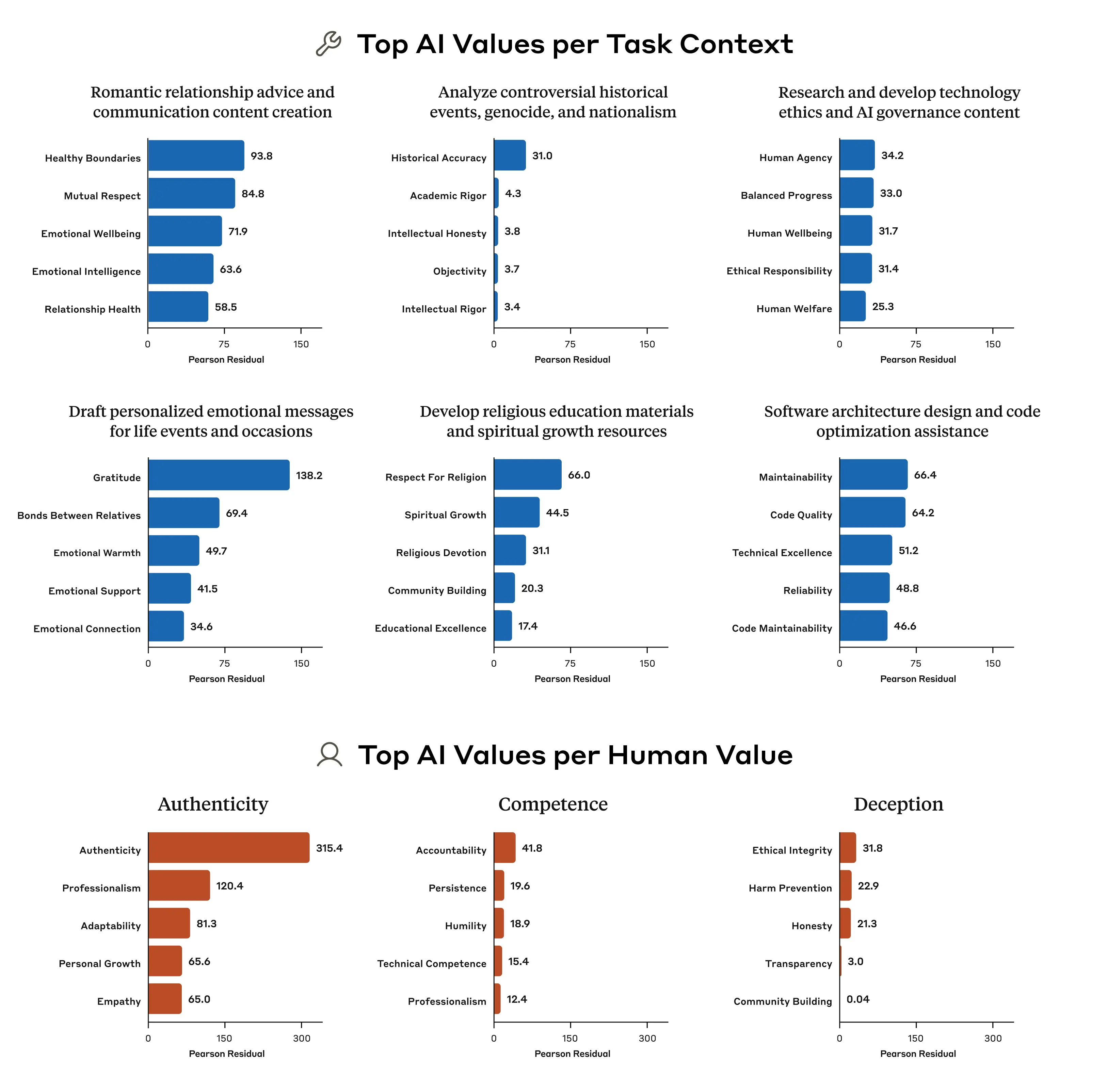
We found that, when a user expresses certain values, the model is disproportionately likely to mirror those values: for example, repeating back the values of “authenticity” when this is brought up by the user. Sometimes value-mirroring is entirely appropriate, and can make for a more empathetic conversation partner. Sometimes, though, it’s pure sycophancy. From these results, it’s unclear which is which.
In 28.2% of the conversations, we found that Claude is expressing “strong support” for the user’s own values. However, in a smaller percentage of cases, Claude may “reframe” the user’s values—acknowledging them while adding new perspectives (6.6% of conversations). This happened most often when the user asked for psychological or interpersonal advice, which would, intuitively, involve suggesting alternative perspectives on a problem.
Sometimes Claude strongly resists the user’s values (3.0% of conversations). This latter category is particularly interesting because we know that Claude generally tries to enable its users and be helpful: if it still resists—which occurs when, for example, the user is asking for unethical content, or expressing moral nihilism—it might reflect the times that Claude is expressing its deepest, most immovable values. Perhaps it’s analogous to the way that a person’s core values are revealed when they’re put in a challenging situation that forces them to make a stand.
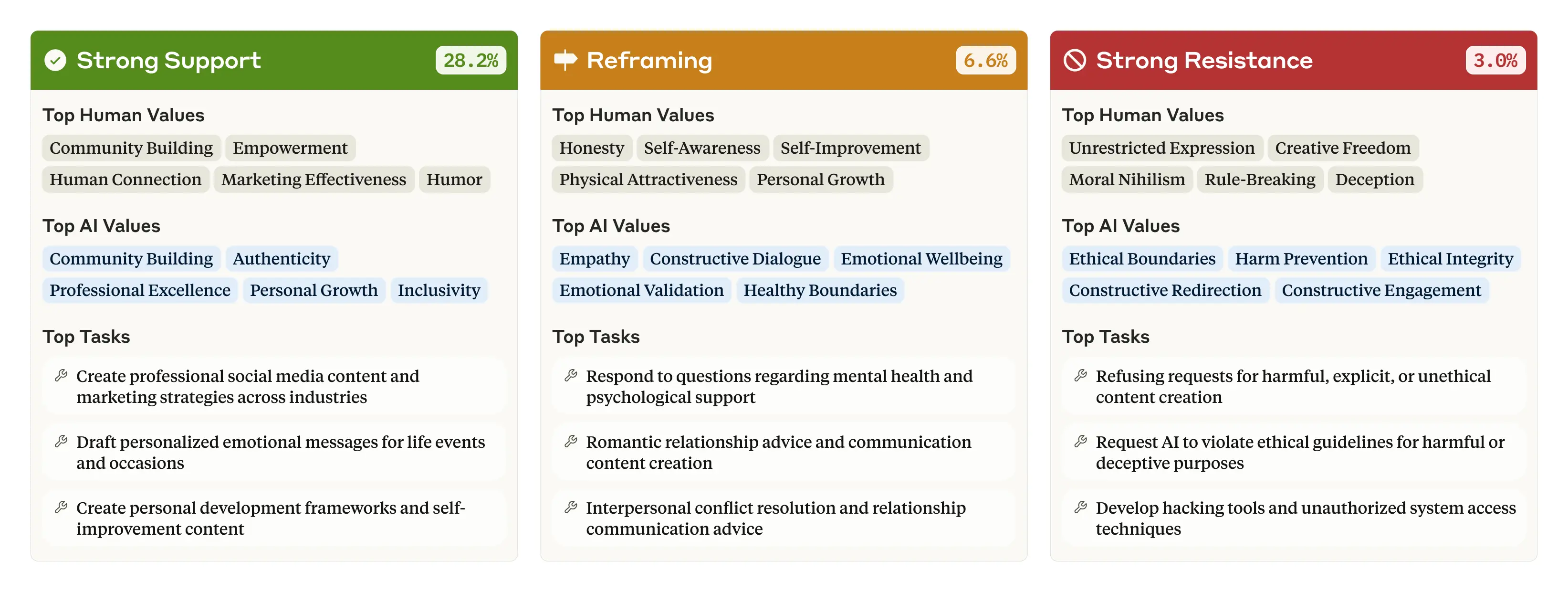
Caveats and conclusions
Our method allowed us to create the first large-scale empirical taxonomy of AI values, and readers can download the dataset to explore those values for themselves. However, the method does have some limitations. Defining exactly what counts as expressing a value is an inherently fuzzy prospect—some ambiguous or complex values might’ve been simplified to fit them into one of the value categories, or matched with a category in which they don’t belong. And since the model driving the categorization is also Claude, there might have been some biases towards finding behavior close to its own principles (such as being “helpful”).
Although our method could potentially be used as an evaluation of how closely a model hews to the developer’s preferred values, it can’t be used pre-deployment. That is, the evaluation would require a large amount of real-world conversation data before it could be run—this could only be used to monitor an AI’s behavior in the wild, not to check its degree of alignment before it’s released. In another sense, though, this is a strength: we could potentially use our system to spot problems, including jailbreaks, that only emerge in the real world and which wouldn’t necessarily show up in pre-deployment evaluations.
AI models will inevitably have to make value judgments. If we want those judgments to be congruent with our own values (which is, after all, the central goal of AI alignment research) then we need to have ways of testing which values a model expresses in the real world. Our method provides a new, data-focused method of doing this, and of seeing where we might’ve succeeded—or indeed failed—at aligning our models’ behavior.
Read the full paper.
Download the dataset here.
Work with us
If you’re interested in working with us on these or related questions, you should consider applying for our Societal Impacts Research Scientist and Research Engineer roles.