How Semgrep delivers AI-powered code security with Claude in Amazon Bedrock
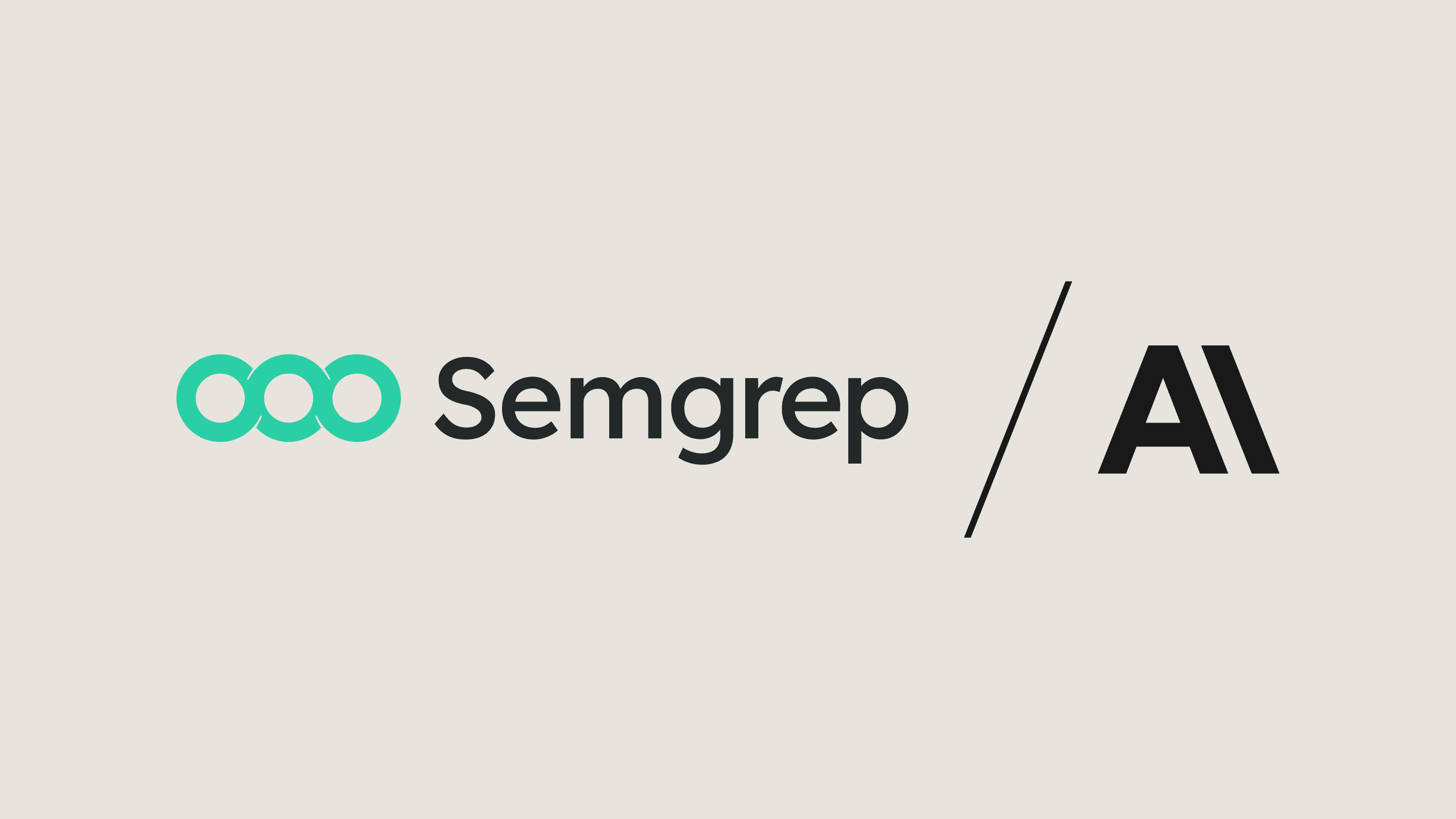
Semgrep, a leading cyber security company, leverages Claude in Amazon Bedrock to power many of its AI-assisted features for customers, helping developers detect, filter, and fix code vulnerabilities more effectively—while minimizing false positives that waste engineering time.
With Claude, Semgrep:
- Confidently labels 20% of security findings as safe to ignore, with a 92% user agree rate and 96% security researcher agree rate
- Achieved 16% higher accuracy identifying false positives than a prior version powered by GPT-4o
- Delivered 17% better performance in component tagging (compared to their previous model, GPT-4o)
- Processes and analyzes thousands of security findings for customers daily
How Semgrep is solving security's false positive problem
Software development teams face an overwhelming volume of security alerts, many of which are false positives that consume valuable time and resources. "Security tools are notorious for generating high volumes of alerts. This is a widespread problem across the industry," said Bence Nagy, Staff Engineer at Semgrep.
Semgrep recognized this pain point and saw an opportunity to apply AI to dramatically reduce alert noise.
Semgrep's fast, deterministic code analysis engine and LLM-friendly rule syntax made it the perfect foundation for AI-powered AppSec—retaining the benefits of precise pattern matching while taking advantage of AI’s ability to understand code context.
Nagy explained their first breakthrough with a practical example: "We tested if an AI model could distinguish between actual leaked passwords in code versus harmless placeholders like 'password=REPLACE_PASSWORD_HERE'.”
Traditional security tools blindly flag both as vulnerabilities, but we discovered that AI could accurately determine which alerts represented genuine security risks and which were false alarms. This initial success proved AI could solve a fundamental problem in security scanning—distinguishing real threats from noise—prompting Semgrep to explore how AI could transform their entire security platform to help teams focus exclusively on legitimate security issues.
Choosing the best model for every task
Semgrep’s AI features rely on a variety of prompt chains and evaluation loops that reference both project-specific inputs (dependencies, prior fixes, dataflow traces) and inputs from Semgrep’s non-AI analyses.
To compare how different models perform on these prompt chains, Semgrep relies on a rigorous evaluation process that ensures they are always using the best LLM across a multitude of security tasks—from filtering false positives to generating accurate remediation guidance.
“Our goal is to make sure developers only see the security issues that matter. We benchmark models against real-world data, constantly monitor performance across over 1000+ customers and let the results guide us,” said Bence Nagy, Staff Engineer at Semgrep.
Claude was unique in that it was a top performing model (top 3) across every single one of Semgrep’s unique, task-specific evals.
"Claude 3.7 Sonnet demonstrated remarkable code analysis capabilities with deeper contextual understanding," said Nagy. "It was the only model that recognized a vulnerability in an auto-generated file, advising developers to fix the generating source rather than editing the output file directly."
In two of the most important evals, false positive detection and component tagging, Claude outperformed GPT-4o, the previous top performing model, by 16% and 17% respectively.
“Operating at the application layer, we see model benchmarking and selection as our responsibility—not the customer’s. While anyone can request the use of a specific model, customers generally trust us to optimize and choose for them on a per-task basis,” said Chushi Li, Product Marketing Manager at Semgrep.
Claude enhances Semgrep’s security capabilities
Semgrep uses a variety of large language models—including Claude—to power features that help security teams cut noise, automate routine tasks, and generate fixes:
- Noise filtering: Analyze security alerts to separate true issues from false positives, reducing alert volume by 20% out of the box, and up to 40% over time.
- Memories: Learns and stores critical, security-relevant context about an environment as users triage findings and fix issues, eliminating future false positives.
- Autofix + Remediation guidance: Suggest one-click code fixes to remediate vulnerabilities, and give developers the context they need to understand and feel confident merging the changes.
- Breaking change analysis: Analyze dependency upgrades for breaking changes, and automatically create upgrade PRs that tell developers if the version bump is safe or if they need to refactor.
- File sensitivity classification: Assess the criticality of files, elevating issues in high-risk components like auth or payments over low-risk ones.
- Rule-writing: Write deterministic Semgrep rules based on instructions in natural language
One of the security tools that developers don’t mind
Semgrep's AI-powered features have improved productivity for security and development teams, and extend beyond noise reduction. Claude's contextual understanding also helps developers implement more comprehensive fixes. "Claude provides additional detail on what developers need to check after implementing a fix and how to adapt other parts of their project," said Nagy. This approach prevents the common problem where fixing a vulnerability breaks existing functionality.
Semgrep's benchmarking approach ensures optimal AI performance for each security task. Nagy explained, "For our noise filtering feature, we've built evaluation suites containing thousands of pre-classified security findings to measure accuracy precisely."
This testing revealed important insights about model configuration, including a counterintuitive discovery: "We found that giving Claude more tokens for 'thinking' actually made it overly cautious about security issues, becoming too conservative in its assessments." These insights allow Semgrep to fine-tune Claude's parameters for security tasks, maximizing its effectiveness for real-world operations.
Claude in Amazon Bedrock: Enterprise security, privacy, and performance
Semgrep frequently works with customers in industries with strict data governance requirements. Amazon Bedrock provides a secure way to access powerful foundation models like Claude within private environments, such as an organization’s Amazon Virtual Private Cloud (VPC).
“Security and privacy are non-negotiable for us and our customers,” said Drew Dennison, Semgrep co-founder. “The ability to access Claude through Amazon Bedrock—within a secure, compliant setup—gives us the flexibility we need without compromising on performance.”
This availability makes Claude a compelling choice—not just for its technical excellence, but for how seamlessly it fits into real-world enterprise environments.
MCP and the future of AI-powered development
Semgrep envisions a future where AI both creates and secures software. "As software development becomes more accessible, less experienced engineers will generate more AI-written code. How do we keep that code secure?" asked Dennison. "We need security tools embedded directly in the AI's generation process, plus verification systems to audit code in the chain of thought."
This vision drove Semgrep to develop an open-source Model Context Protocol (MCP) tool that enables AI assistants like Claude to scan their generated code for vulnerabilities before delivering it. Since Semgrep’s core SAST engine is fast, transparent, and source-based, an MCP server makes it possible for LLMs to dynamically call Semgrep as a tool. Nagy said, "We want to create a world where you can build an entire project with AI and be confident it's secure and functional."
The company is also developing organizational memory features to enhance contextual security. "We're building systems that learn from developer comments and decisions to understand non-obvious facts about a codebase," explained Nagy. By capturing this institutional knowledge, security assessments become more intelligent over time.
Through these innovations, Semgrep aims to ensure AI accelerates development while maintaining security—benefiting the entire software industry with both speed and safety.